Oscar Pistorius trial shows the importance of data management
Oscar Pistorius’ trial has been dubbed the ‘trial of the century’ and is receiving a lot of media attention. A young woman has died, under tragic circumstances and the fate of her killer lies in the evidence, the prosecutions ability to create a picture from that evidence, and the ability of the defence to create reasonable doubt. The same concept can be applied to data management within an organisation.
Typically, we think of data as information captured in a database. The evidence used in this trial serves as a reminder that data is simply raw information and can take many forms.
A court case presents evidence - data - and draws conclusions based on this data. This can be likened to the decision-making process of any business. Ironically, in a court case, the prosecution and the defence are each pushing for diametrically opposed conclusions – innocence or guilt, using the same data.
While the merits of the case are for the court to decide, what has been interesting is the legal process itself, and how the opposing counsel is applying data management principles to support their desired outcomes.
Indeed, the outcome of this case could depend on the data management principles of data governance and data quality.
Subscribe content preview
To continue reading: Log-In above or Subscribe now.
Want the full story?
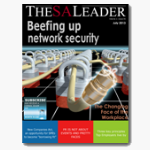
SUBSCRIBE NOW
Get The SA Leader the way you want it
- One Year Digital Subscription - R320
- 10 Issues Print Subscription - R580
- One Year All Access - Just R900 Best Deal!
Print Magazine + Digital Edition + Subscriber-only content on SALeader.co.za
If you are already a subscriber, please Log-In using the Log-In button found on the top right of the site!
Plan for a successful data migration
Data integration and migration challenges arise every time an organisation moves to a new data system, or wishes to combine multiple data systems, either internally or as a result of a merger. This is a significant undertaking, which, if it fails to meet expectations, inhibits a business’ ability to function effectively. Yet, surveys indicate that more than 80% of all data integration projects fail. There are several reasons for this failure, but the most commonly cited issue is a lack of understanding of the extent and nature of problems that were to be experienced within the data itself. Data quality is critical to the success of any data integration and migration initiative, and organisations leave this aspect to the last minute at their peril.
Adopting a Lean approach to data governance
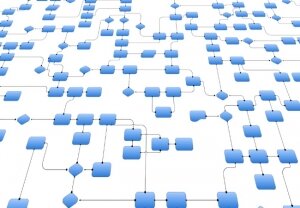
Data governance is fast becoming a priority on the business agenda, in light of regulations and guidelines such as King III, which outline recommendations for corporate governance of which data governance forms a critical part. However, data governance can be a challenging and complex task. Adopting a Lean approach to data governance can help businesses to eliminate wasteful data governance activity and promote efficiencies.
BI trends in retrospect: first call to board the EIM train

At the beginning of 2012 the research firms and pundits proposed numerous trends for the business intelligence (BI) world. Statistical analytics, BI in the cloud, mobile BI, in-memory analytics, agile BI, and big data were among the common technologies, methodologies and processes purportedly top of mind.
In essence all came true in South Africa, where many business executives and IT leaders did investigate some or even all of the top issues, but not all were deployed. Investigations into employment highlighted some major issues that have held the progress of BI in check and left some leaders querying suitability and just how to go about implementing the technologies.
Assessing your data quality – going back to basics
We all understand that quality information is an enabler for cost cutting, risk reduction and revenue enhancement. Yet, companies have different approaches to managing the corporate information assets, ranging from ad hoc, tactical projects to meet specific goals, to strategic imperatives that seek to embed data quality principles across the corporate architecture.
This makes it difficult to know where to start, what is effective, or whether you are on track or not for success in meeting the data management challenge.
For many organisations data management remains a reactive process, with individual project teams or business areas identifying specific data issues that are impeding the ability of the project to achieve a specific goal. Short-term measures may include tactical data cleansing initiatives, with or without a tool, and typically do not take corporate standards and goals into account.
Reaching a mature state requires a metamorphosis from this chaotic approach to more proactive approaches that incorporate data quality metrics, standards, shared master data and data stewardship. An enterprise driven focus on data management requires data management principles to be embedded in the corporate culture - being driven both top down and bottom up.
Just a caterpillar does not become a butterfly in one step, it is important to build upon early successes in a sustainable way to reach data management maturity. The starting point is being able to understand the state of your data and once this is established, planning how to improve this is the next logical step. From here, identifying opportunities for incremental improvement will allow you to maximise the value of your information with benefits such as lowered costs, improved efficiencies and customer retention to mention a few.
A crucial success factor is to appoint custodians of the data that take responsibility and most importantly, are accountable for the state of the data. Many organisations have failed to achieve data integrity due to the fact that IT blames business, business blames IT and the end user blames their line manager. Data has no boundaries and is an integral part of each and every component within the business, whether it is sales, finance or operations. Setting the company up for successful data quality means that responsibility must be clear across the entire organisation, and that the consequences of non-delivery are also clear.
Once this step is addressed, it is pointless embarking on a data quality exercise or project if there are no measurements in place. It makes sense to create a ‘baseline’ of the quality of your data (which is usually established with a survey and a data audit) and then key value indicators (KVIs) should be established in order to measure the improvement and success of the data quality initiative. These baselines should be linked to the business impact of failing data quality – there is no point in trying to address data quality issues that have no commercial impact on your business.
In order to fully realise the benefits of a data quality exercise, having the right tools is another fundamental. Many companies are lulled into the false sense of security that their ‘stack’ solution that incorporate data cleansing will suffice but more than often, the functionality is limited. Specialist tools are purpose built and therefore provide richer features and functionality. However, it is also important to note that technology alone is not the panacea to a business’ data quality woes. It is recommended that a data quality specialist assists with the project and in some instances, it is better to outsource the project or initiative to a specialist. They deal with data quality issues on a daily basis which ultimately means they have more experience and insight into some of the trickier issues that need to be dealt with.
In order to tackle the first step of establishing the data of your data, take this free survey, , to assess the effectiveness of your approach against those taken by over a thousand IT and business professionals across the globe.
Big Data – what’s the big deal and why is data quality so important?

In today’s world volumes of data are rapidly expanding, and the nature of this data is more varied and complex than ever before. While this concept of Big Data brings with it numerous possibilities and insights for organisations, it also brings many challenges, particularly around the management and quality of data. All data has potential value if it can be collected, analysed and used to generate insight, but in the era of Big Data new concepts, practices and technologies are needed to manage and exploit this data, and data quality has become more important than ever.
Big Data has three characteristics, namely volume, variety and velocity. Data today is growing at an exponential rate, with around 90% of all digital data being created in the last two years alone and somewhere in the region of 2.5 quintillion bytes of data being generated each and every day. Data is more varied and complex than ever, consisting of a mixture of text, audio, images, machine generated data and more, and much of this data is semi-structured or unstructured. This data is often generated in real-time, and as a result analysis and response needs to be rapid, often also in real-time. This means that traditional Business Intelligence (BI) and data warehousing environments are becoming obsolete and that traditional techniques are ill equipped to process and analyse this data.
When it comes to generating Big Data there are multiple sources, some widely known and some that organisations rarely think about. Photographs, emails, music downloads, smartphones, video sharing and social networks account for a large proportion of this data, and are well known sources. But in today’s digital world there are many other places that data may come from, including point of sale devices, RFID chips, recording and diagnostic tools in aeroplanes, cars and other transport, manufacturing, and even scientific research.
All of this machine generated data, along with Internet and socially generated data, can potentially be a source of insight and intelligence. Big Data has the potential to help organisations gain a better understanding of customer and market behaviour, improve knowledge of product and service performance, aid innovation, enhance revenue, reduce cost, and enable more rapid, fact-based decisions which can enhance efficiency, reduce risk and more. The sheer volume of Big Data presents a massive potential pitfall however, and the challenge lies in being able to identify the right data to solve a business problem or address an opportunity, and in the ability to integrate and match data from multiple sources.
In order to leverage the potential of Big Data, it is vital for organisations to be able to define which data really matters out of the explosion of information, and assure the quality of internal data sources to ensure accuracy, completeness and consistency and enable this data to be matched with that from external sources. It is also important to define and apply business rules and metadata management around how the data will be used, and a data governance framework is crucial for consistency and control. Processes and tools need to be in place to enable source data profiling, data integration and standardisation, business rule creation and management, de-duplication, normalisation, enriching and auditing of data amongst others. Many of these functions need to be capable of being carried out in real-time, with zero lag.
Data quality is the key enabler when it comes to leveraging actionable insight and knowledge from Big Data. Poor data quality around the analysis of Big Data can lead to big problems. Consolidating various sources of data without data quality will create a mess of conflicting information, and conducting analytics on data without first assuring its quality will likely lead to the wrong results, poor decision making, and other negative consequences, including lack of compliance which has massive legal implications. The three V’s of Big Data, namely volume, velocity and variety, need to be met by a fourth V – validity – in order to harness this data for the benefit of an organisation and its customers.
While the concept of Big Data may have been over-hyped, the reality is that it is here, and it will continue to grow in volume, velocity and variety. While it is currently an immature concept, as it begins to mature data will increasingly be viewed as a strategic business asset and data skills will be increasingly valued. Big Data reflects the expectations and needs of the emerging generation, and businesses would do well to pay attention to this, and ensure that their data quality initiatives are up to speed to ensure they can leverage the potential value of the Big Data phenomenon.